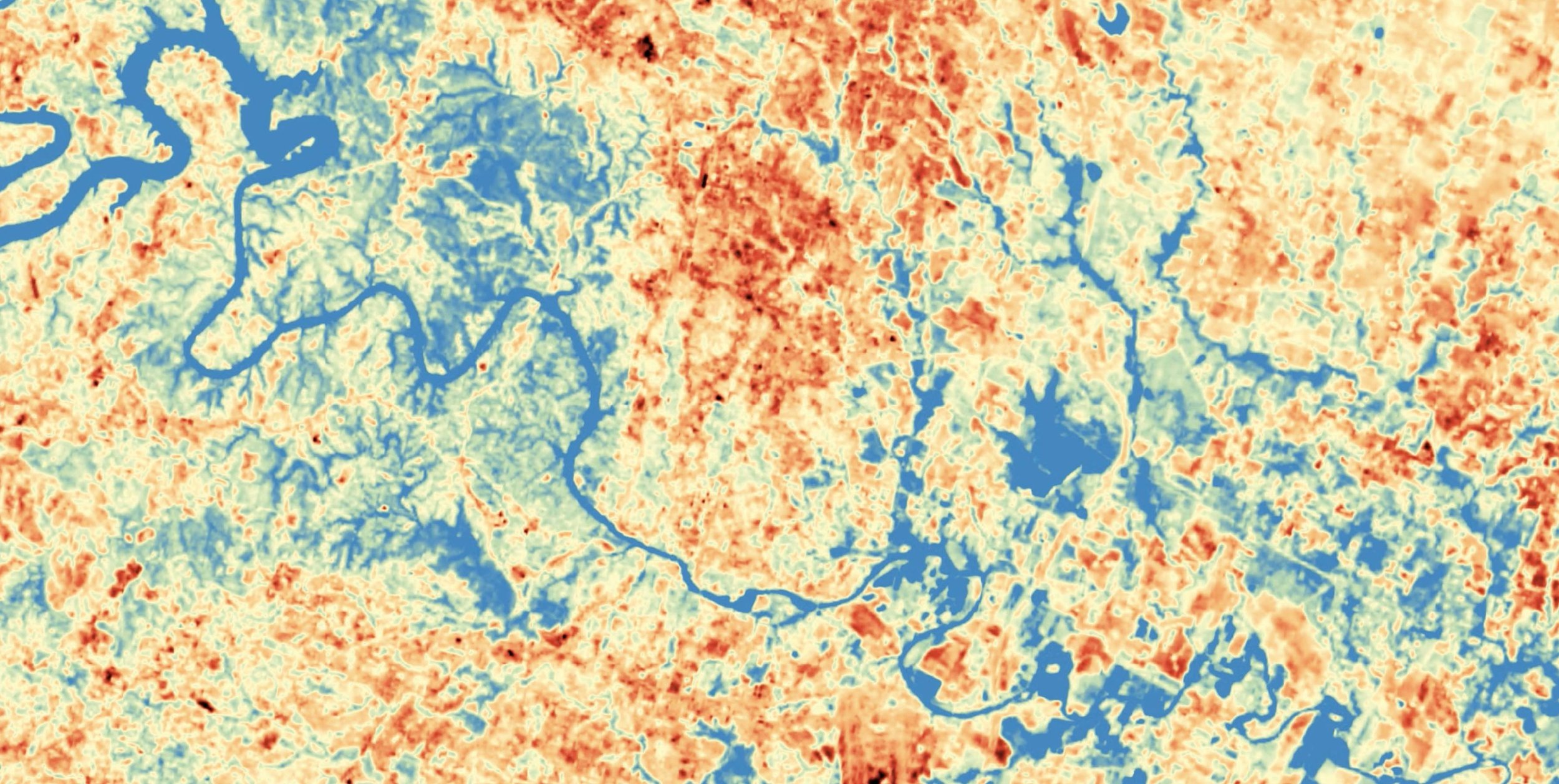
Artificial intelligence
Working with commercial clients and US government agencies to provide computer vision and machine learning expertise, including large-scale synthetic data generation.
Synthetic data generation
Creating high quality, bespoke data at scale
Machine learning algorithms for computer vision tasks require large quantities of high quality representative annotated data. Synthetic data provides an essential bridge when working with non-traditional and higher-dimensional data types, data that is expensive to capture, or where labeling and annotation tasks are impractical - such as for 3D XCT data.
Synthetik develops techniques for generating automatically annotated synthetic computer vision data at volume and low cost to supplement real-world datasets, allowing the acceleration of machine learning model development across a range of different applications in the computer vision domain.
Examples of Synthetik’s work include the creation of synthetic waveform velocity data, hyperspectral infrared images, 2D and 3D baggage scans and millimeter wave scans. Synthetik has been recognized with Nvidia and Reverie as a global vendor of synthetic data.
Synthetik AI | Segmentastic
An automated AI-based platform for 3D object recognition, segmentation, annotation, and synthetic data generation
Segmentastic is an ‘on-the-fly’ machine-assisted annotation capability – as new unknown objects are scanned, identified, and classified, the system will ‘learn’ the new object, thus increasing system accuracy and reducing false positives over time.
A collaborative annotation platform for both 2D images and 3D scans, Segmentastic allows teams to jointly create bounding box and label annotations for objects in 2D and 3D media for use in later machine learning projects. Suitable for multiple applications, Segmentastic has already been deployed to the TSA (U.S. Transportation Security Administration) for scanning passenger baggage.
Physics-Informed Neural Networks (PINNs)
Leveraging governing physical equations to enhance neural networks
PINNs are able to generate robust, accurate models with less data by leveraging governing physical equations to enhance the information content available. Synthetik successfully uses synthetic and real-world data to train and test various PINN architectures to quickly establish the feasibility of approaches to solving real-world engineering problems.
For example, we have utilized PINNs in the design of models used for building design in coastal regions affected by high winds, subterranean shipyard mapping, high-risk flood areas, and urban heat islands, etc.
Custom solutions to fit your needs
Not seeing what you’re looking for? We create solutions for a variety of needs in insurance, global environment, energy, and defense & security. Reach out and we’ll be in touch.